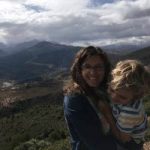
Kimmy Gustafson
The roots of predictive policing can be traced back to the 1920s when the Chicago School of Sociology researched parole recidivism. They then used this data to factors that would correlate to the prediction of future crimes.
In the 1990s, this was taken a step further when law enforcement agencies began using data-driven approaches to enhance their crime prevention strategies. Pioneered by the New York City Police Department, the CompStat system emerged as an early tool that utilized statistical data to map crime patterns and trends. This system allowed for more proactive and targeted policing, which led to significant reductions in crime rates. However, it also raised concerns about the potential for disproportionate impacts on certain communities.
The incorporation of artificial intelligence (AI) into predictive policing represents a more recent evolution, beginning in earnest in the 2010s. AI algorithms have been developed to analyze vast data sets, including historical crime data, social media activity, and other relevant information, to predict where crimes are likely to occur and identify potential offenders. While proponents argue that these advancements can further enhance the efficiency and effectiveness of policing, critics caution against the risks of bias, privacy infringements, and ethical dilemmas.
Predictive policing is becoming increasingly prevalent across various domestic and international jurisdictions. In the United States, major cities like Los Angeles, Chicago, and Atlanta have adopted predictive policing technologies to augment their traditional law enforcement methods. “It is all over the place, in different ways. It’s becoming popular in big cities, but even smaller cities use predictive software or things like the strategic subjects list. Often smaller enforcement agencies collaborate with larger agencies’ data collection efforts, creating really broad networks,” says Dr. Andrea DaViera, community psychologist, community organizer, and educator at the University of Illinois, Chicago.
While these programs are touted to have benefits, they have unintended consequences, too: “These systems are impacting people in communities. Surveillance might not seem particularly harmful, but, when we see it put into practice, it’s both very carceral and helps shimmy people into the criminal legal system,” notes Dr. DaViera. “What is really the purpose of this thing? Is it to try to create safety? Because there’s a lot of evidence that it doesn’t create safety. So what can be a more creative way to think about creating safety, reducing violence, and promoting healthy relationships?”
Keep reading to learn the answers to DaViera’s questions and more.
Dr. Andrea DaViera is a community psychologist, community organizer, and educator. She received her PhD in community and applied developmental psychology from the University of Illinois Chicago in 2024.
Dr. DaViera has two broad research interests: 1) how racism and the Prison Industrial Complex (PIC) impact health and wellness through creating the conditions for interpersonal violence and structural violence, and 2) how individuals and communities collectively resist and thrive despite racism and oppression. She aims to create systems-level impact through pairing research and community organizing.
To understand the issues of predictive policing, it is first necessary to understand what it is: “Law enforcement takes historical data that they’ve accumulated through their own surveillance systems including crime data, arrest reports, geographic and socioeconomic information and try to predict when a crime is happening, who’s committing crimes, or where it might happen,” explains Dr. DaViera.
Deductions are made based on the data using algorithms and AI. Police departments use these conclusions to allocate resources, make strategic decisions, and identify individuals for surveillance or intervention.
One prominent example of predictive policing technology is the Strategic Subjects List (SSL), also known as “heat lists.” The SSL is an algorithmic tool designed to identify individuals who are deemed at high risk of either being involved in a violent crime or becoming victims themselves. This methodology, prominently implemented in cities such as Chicago, processes a variety of data points, including criminal records, gang affiliations, social network connections, and victimization histories, to compile a list of potential subjects.
“The list was historical data. It included arrest records and anyone who had interacted with the law enforcement system. Apparently, those who had simply been fingerprinted also found themselves in this data set. Then, using an algorithm, it deduced a score of whether that person would be a victim or a perpetrator of gun violence. Really interestingly, that algorithm did not distinguish whether they would be the shooter or the person being shot,” says Dr. DaViera.
The idea behind predictive policing is to apply data-driven methods to law enforcement to prevent and reduce crime. Proponents argue that these technologies can help allocate resources more efficiently, predict potential hotspots for criminal activity, and identify individuals who may benefit from intervention or support services. In theory, this could lead to a reduction in crime rates and improved community safety. However, not only does predictive policing now reduce offenses, but it has also been shown to be incredibly biased.
Despite the theoretical advantages, predictive policing introduces a host of significant concerns that cannot be overlooked. Critics argue that these systems often perpetuate existing biases, leading to disproportionate targeting of marginalized and minority communities. Additionally, the reliance on historical data, which may already be tainted by biased policing practices, further exacerbates these inequalities.
To illustrate this, Dr. DaViera references a program that was utilized in Chicago and other major cities: “There is a service called ShotSpotter/SoundThinking that places microphones all over the city to pick up sounds of gunshots and then alert police to go to that place. However, an evaluation of it found that many of those alerts didn’t lead to any violent gun situation. So, what were the microphones picking up on? They’re picking up on someone’s car, making a loud sound. They’re picking up on the train. They’re picking up on all sorts of sounds,” she says. “ But it creates an alert just the same and brings the police into a neighborhood, ready for a gun show. They are on alert for violence, which could lead to more violence.”
In fact, a survey conducted by the MacArthur Justice Center of Northwestern University Law School found that this technology sent police on 40,000 dead-end deployments in 21 months. “There’s no evidence showing that this is actually reducing crime in any way. And then the other thing is, through a Freedom of Information request, they have uncovered that the microphones are predominantly placed in black and Latino neighborhoods,” says Dr. DaViera.
Predictive policing strategies that rely on analyzing existing data sets also face challenges: “There’s a predisposition for Black and Brown people to be in the data set. Primarily because they’re over-arrested. An evaluation study of the strategic subjects list found that if you were in the data set, it only predicted that you would be arrested again. It creates a cycle where, if you’re arrested, you might be more likely to be arrested because you might be a Black or Latino person, or even just being male,” notes DaViera. “If you are on the list, you’re more likely to be interacted with by the police. And then, because of that interaction, you’re more likely to be arrested. Which leads to more future surveillance.”
According to the Stanford Open Policing Project, you are four times more likely to be stopped by the police if you are Black versus white and two times more likely to be arrested.
Not only is the data racially biased, but the algorithms used in predictive policing can incorporate substitute variables for race, further perpetuating the problem. These use proxies, such as zip codes, socioeconomic status, and historical interaction with law enforcement, often correlate strongly with race: “The Strategic Subjects List algorithm had a number of different variables over the years to create a risk score. They don’t use variables like race, but what they do use are variables like being involved in a previous shooting incident, supposed gang affiliation attempts, or previous other interactions with the criminal legal system. All those things could be considered racial proxies,” says Dr. DaViera. “Those variables are so racially biased, which makes the algorithm racist.”
Relying on such proxies can have far-reaching consequences. Not only do they sustain the cycle of over-policing in marginalized communities, but they also erode trust between law enforcement and the public. This dynamic complicates efforts to foster community engagement and cooperation, essential in creating safer and more just societies.
Given the numerous concerns surrounding predictive policing, it is essential to consider alternative approaches that prioritize community well-being and address underlying issues instead of relying solely on technology: “[I called for diverting] resources towards different ways of addressing safety and crime and violence. The massive amount of resources and human capital that goes into maintaining those systems are actually just quite racist, and when you try to make them more efficient, you’re actually just making the racism more efficient,” says Dr. DaViera
She continues, “We need to move away from surveillance and towards more observational policies. You can observe violence in a neighborhood without surveilling people…Observing a neighborhood could be as simple as walking through it. It can include mapping out where the resources are, where the areas of danger are, and where places are where people are able to have a safe space,” advocates Dr. DaViera.
“Another option is shrinking the size and scope of what police are supposed to do—that way 911 calls that are nonviolent are diverted to emergency and healthcare workers that are not police so they don’t show up with weapons and a badge. Instead, they show up with an ambulance and a social worker.”
Investing in community-based solutions that address the root causes of crime, such as poverty and lack of access to resources, can also create safer environments. This approach shifts away from punitive measures and toward a more holistic understanding of what creates safety for marginalized communities.
Kimmy Gustafson
Kimmy Gustafson’s expertise and passion for investigative storytelling extends to the world of forensics, where she brings a wealth of knowledge and captivating narratives to readers seeking insights into this intriguing world. She has interviewed experts on little-known topics, such as how climate crimes are investigated and prosecuted, and has written for ForensicsColleges.com since 2019.
Kimmy has been a freelance writer for more than a decade, writing hundreds of articles on a wide variety of topics such as startups, nonprofits, healthcare, kiteboarding, the outdoors, and higher education. She is passionate about seeing the world and has traveled to over 27 countries. She holds a bachelor’s degree in journalism from the University of Oregon. When not working, she can be found outdoors, parenting, kiteboarding, or cooking.